Researchers estimate more accurately the short-term solar irradiance
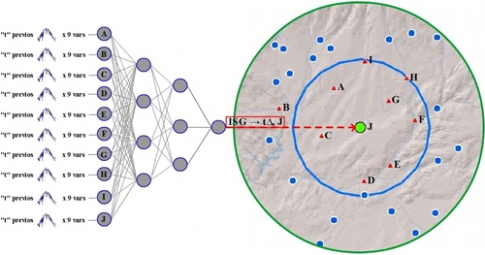
Simplified representation of the developed RNAs models. Credits UPM
Researchers from Universidad Politécnica de Madrid and Nicaraguan Institute of Territorial Studies predict with minor error rate the short- term Global Solar Irradiance (GSI) using Artificial Neural Networks (ANN).
The study, carried out by a team of researchers from Universidad Politécnica de Madrid (UPM) and Nicaraguan Institute of Territorial Studies (INETER), introduces a new methodology based on observations made in parallel by neighbouring sensors and values for different variables (temperature, humidity, pressure, wind and other estimates). Experiments were carried out using Artificial Neural Networks with different architectures and parameters in order to determine which of these generated the best predictions for the various time frames studied.
Results allowed researchers to develop models that predict short-term Global Solar Irradiance with error rates less than 20 percent. This can be useful for companies that manage both photovoltaic solar energy plants and solar thermal plants to estimate the production capacity of their installations.
A big challenge of modern society is the efficient use of natural resources and environmental impact minimization due to the increased demand and consumption of energy. Thus renewable energy, especially solar energy has become a long term solution with greater potential, more sustainable and less impact.
In particular, photovoltaic solar energy can be connected to the transport and distribution network but it requires that supply and demand for energy are adequately managed. Likewise the operators of solar energy systems need to know in advance the solar radiation that will receive their installations throughout all stages (planning, construction and subsequent operation). Therefore, the prediction of the Global Solar Irradiance (GSI) within a few hours and with the minor error rate possible is required to estimate the expected energy production.
Various methods are known to estimate the Global Solar Irradiance such as numerical predictions based on locations and time complemented with diverse correction models, methods based on satellite images that record the cloudiness and estimate the losses in the ideal model, other models based on time series and others based on Artificial Intelligence. Each method has advantages and disadvantages. For instance, the satellite-based prediction has proved to be universal since it provides estimations for large geographical areas. However it has the drawback of the availability of such images for certain regions of the planet and the pre-processing of the images, among others.
In the case of predictions based on numerical methods, the dynamics of the atmosphere are estimated in a realistic way through the assimilation of data. However, they guarantee the general stability of the forecast on local meteorological events. So far, the methods based on Artificial Intelligence only use the endogenous input variables which are associated to the place of the prediction.
The study carried out by UPM and INETER researchers focused on the hypothesis that it was possible to improve the short-term forecast of the Global Solar Irradiance through the generation of models based on Artificial Neural Networks using up to 900 inputs that show the evolution of variables in a nearby spatial"“temporal context.
The image above is a simplified representation of one of the models developed to predict the Global Solar Irradiance in the future using as input data the registered observations (space-time) at the selected areas (from "A" to "J" in the graph). We can see that each location provides 9 different variables.
The research results show the capacity of the developed models based on Artificial Neural Networks to identify both linear and non-linear relationships among the different variables. Researchers say "this has allowed us to predict the short-term Global Solar Irradiance with a significant forecast skill and Normalized Root Mean Square Error less than 20 percent compared to the rest of models based on Artificial Neural Networks. Besides, the developed methods have allowed us to identify a relationship among the predictions via a sliding window of time of 1-3 hours and 4-6 hours regarding the reference distance of 55km (image represented with a blue circle). This can lead to a research line to use diverse reference distances for different prediction slider windows".
These results have diverse applications, for instance, on companies that manage both photovoltaic solar energy plants and solar thermal plants to estimate the production capacity of their installations as current legislation requires and the operators of national electrical systems. Both examples can use these methods to achieve their goals more efficiently: maximize return on investment and adjust the curve of demand and supply.